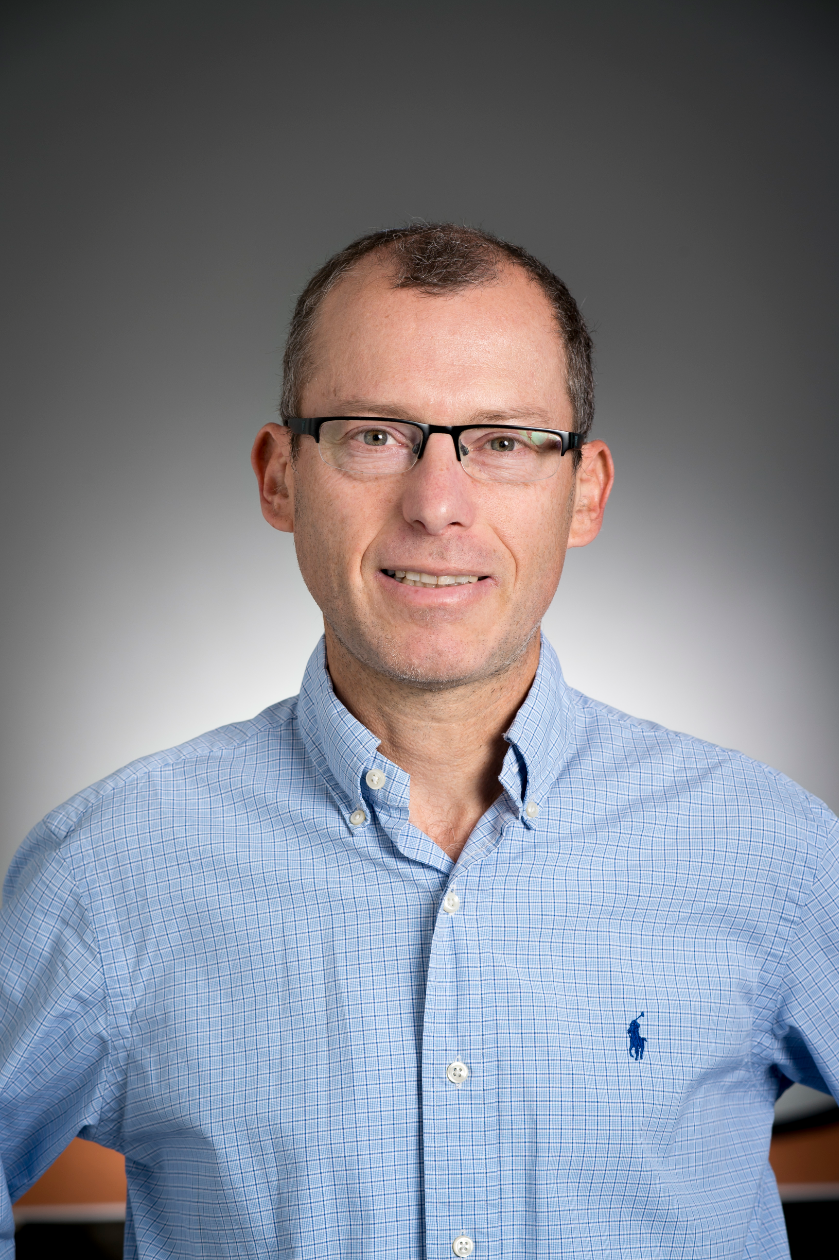
Ziv Bar-Joseph
Carnegie Mellon University
Machine Learning Department
Computational Biology Department
Title: AI / ML in big pharma
Abstract:
While much of the cutting edge work in omics data analysis and modeling is still mainly in academia, biotechs and pharma are very advanced, and in some cases leading, in areas related to molecular design and clinical data analysis. I have been leading the AI / ML work for R&D at one of the largest pharma companies for almost two years and will share some of the methods we have been developing and using to address computational challenges across all stages of the drug discovery and development process. I Will also try to share some of the lessons I have learned over this period.
Short-Bio:
Ziv Bar-Joseph is VP and Head, R&D Data and Computational Sciences at Sanofi and the FORE Systems Professor of Machine Learning Department and Computational Biology in the School of Computer Science at Carnegie Mellon University (CMU). Dr. Bar-Joseph received his PhD in computer science from MIT in 2003. His work is focuses on the development of machine learning methods for the processing, analysis, visualization and modeling high throughput biological data. He has recently worked on the analysis and modeling of single cell data from several modalities and on methods for integrating this data with bulk interaction data to reconstruct networks within and between cells. Dr. Bar-Joseph is involved in a number of national efforts focused on using single cell data to create 3D reference human maps. He led the Computational Tools center for the NIH HuBMAP program and is a PI in one the SenNet Data Coordination Center. He was also the director of the Joint CMU Pitt PhD Program in Computational Biology (CPCB). Dr. Bar-Joseph is the recipient of the Overton prize, the annual award of the Intentional Society for Computational Biology (ISCB) and the NSF CAREER award. He is also the recipient of several best papers awards including at the top computational biology conferences, Recomb and ISMB.

Guoping Zhao
Shanghai Institutes for Biological Sciences, Chinese Academy of Sciences, China
Title: Standardized Integration and Intelligent Governance of Biomedical Data
Abstract:
In recent years, the rapid growth in types and scales of biomedical data has significantly transformed our research paradigms. However, the standardized integration and governance of multi-source heterogeneous data remain key challenges in maximizing data value. In human phenome research, we utilized the Phenotype Assembly Framework (PAF) to achieve multi-dimensional, cross-scale precise descriptions of phenotypes through 13 categories of features and qualifiers. Based on PAF, we developed standardized Phenome Data Elements (PhenoDE) for 22 human phenome platforms, with the majority successfully mapped to nationally recognized data standards and transferable to large cohorts such as the UK Biobank. Leveraging the LLM-based PhenoAgent, we identified outliers and characterized data distribution in international real-world data. Similarly, we standardized and governed metadata from Chinese clinical records, forming Clinical Research Essential Data Elements, and utilized LLM to structure and standardize real-world data. In microbiome research, LLM-driven governance of omics data sample information significantly improved data completion rates. Through these efforts, we have leveraged LLM to govern data, providing high-quality corpora for vertical domain large models.
Short-Bio:
Zhao Guoping, molecular microbiologist, born in Shanghai in 1948, is a professor of Institute of Plant Physiology and Ecology (IPPE), Shanghai Institutes for Biological Sciences (SIBS), Chinese Academy of Sciences (CAS). Currently, his major administrative positions are executive director of the Chinese National Human Genome Center at Shanghai (CHGCS) and director of the National Engineering Center for BioChip at Shanghai. He is also a professor and director of the Department of Microbiology and Microbial Engineering, School of Life Science, Fudan University. He obtained his B.S. of Microbiology from Fudan University, Shanghai, China in 1982 and Ph.D. of Biochemistry from Purdue University, W. Lafayette, Indiana, USA in 1990.
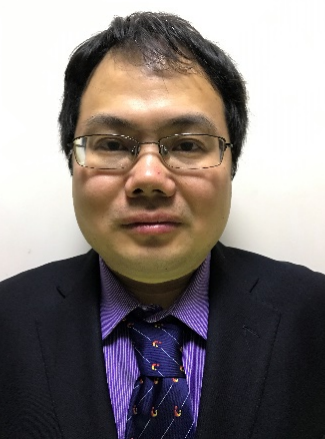
Guoqing Zhang
Shanghai Institutes for Biological Sciences, Chinese Academy of Sciences, China
Title: Standardized Integration and Intelligent Governance of Biomedical Data
Abstract:
In recent years, the rapid growth in types and scales of biomedical data has significantly transformed our research paradigms. However, the standardized integration and governance of multi-source heterogeneous data remain key challenges in maximizing data value. In human phenome research, we utilized the Phenotype Assembly Framework (PAF) to achieve multi-dimensional, cross-scale precise descriptions of phenotypes through 13 categories of features and qualifiers. Based on PAF, we developed standardized Phenome Data Elements (PhenoDE) for 22 human phenome platforms, with the majority successfully mapped to nationally recognized data standards and transferable to large cohorts such as the UK Biobank. Leveraging the LLM-based PhenoAgent, we identified outliers and characterized data distribution in international real-world data. Similarly, we standardized and governed metadata from Chinese clinical records, forming Clinical Research Essential Data Elements, and utilized LLM to structure and standardize real-world data. In microbiome research, LLM-driven governance of omics data sample information significantly improved data completion rates. Through these efforts, we have leveraged LLM to govern data, providing high-quality corpora for vertical domain large models.
Short-Bio:
Dr. Zhang Guoqing is the Deputy Director of the Biomedical Big Data Center at the Shanghai Institute of Nutrition and Health, as well as the Deputy Director of the Key Laboratory of Computational Biology, Chinese Academy of Sciences. He also serves as the Deputy Chair of the Special Committee on Biological Data Resources under the China Bioinformatics Society (in preparation). Dr. Zhang has led the development of big data platforms in several major national and regional projects, including the National Precision Medicine Initiative, the CAS Pioneer B Project, and a major scientific instrument development project supported by the National Natural Science Foundation (departmental recommendation). He has established a "1+2+3" big data technology framework: 1 multi-omics data convergence platform (NODE), 2 interactive multi-omics data analysis platforms (ViPMAP and iMAC), and 3 standardized and integrated platforms for human phenotypes, natural population cohorts, and clinical research big data. Dr. Zhang has published over 80 papers in journals such as Nature Microbiology, National Science Review, Nucleic Acids Research, Nature Protocols, and Briefings in Bioinformatics. He holds one patent and has more than 100 software copyrights.
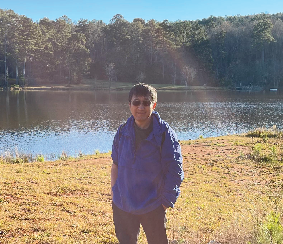
Ying Xu
Southern University of Science and Technology, China
Title: Studies of cancer in the big data age
Abstract:
The ability of generating large quantities of omic data of cancer tissues provided unprecedented opportunities for cancer researchers to study cancer evolution in a realistic setting. Here I present a computational study of cancer drivers and key mechanisms at the chemistry imbalance level guided by information derived from omic data analyses and modeling. Cancer generally has considerable changes in their cellular chemical conditions such as the pH and the O2 level, which result in changes in the cellular physical conditions, including the membrane potential and the intracellular polarity. These changes will profoundly alter the kinetics and thermodynamics of cellular chemical reactions, leading to the so-called metabolic reprogramming (MR) at a systems level, created by stress-induced genetic mutations and epigenetic alterations for survival. The affected cells may have to make drastic and fundamental changes, such as the significant simplification of their polarity system (which defines what a cell can and cannot do) via mutations as in cancer, to generate sustained metabolic exits for the newly created MRs, which give rise to specific phenotypes of a cancer. To elucidate the operating principles of cancer, or specifically how biological functions encoded in our genome behave in a fundamentally novel physicochemical microenvironment, we need to have a new research framework, which requires, at least, consideration of biology at the basic chemistry level.
Short-Bio:
Ying Xu is a Chair Professor in the School of Medicine, Southern University of Science and Technology, China since January 2023. He is also a Cheungkong Scholar Chair Professor (2008 - ) and Qianren Chair Professor (2012 - ). Before that, he was a Regent Professor and the Georgia Research Alliance Eminent Scholar Chair in the Department of Biochemistry and Molecular Biology, the University of Georgia (2003 – 2022/12) and the Founding Director of the Institute of Bioinformatics, the University of Georgia, USA (2003-2011). He is an AAAS Fellow and an IEEE Fellow. He has been a computational biologist since 1993 when he joined the Oak Ridge National Laboratory to take part in the Human Genome Project, where he worked for ten years and moved up the career ladder from a research associate to a senior staff scientist and group leader. He has published over 400 research papers and five books, including the world's first monograph “Cancer Bioinformatics”. His H-Index is 72 with ~21000 citations in scholar.google. He received his Ph.D. in theoretical computer science from the University of Colorado in 1991 and earlier degrees from Jilin University, China.
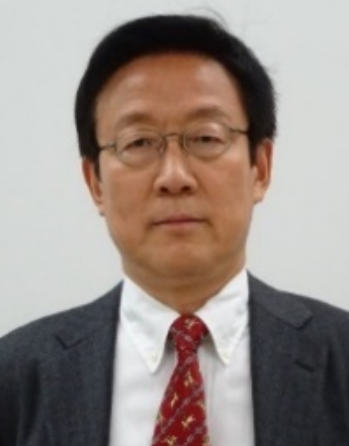
Ning Zhong
Maebashi Institute of Technology, Japan
Title: Web Intelligence Meets Brain Informatics: Building the Foundations for Future Intelligent Societies
Abstract:
This keynote explores the transformative fusion of Web Intelligence and Brain Informatics, focusing on how their convergence is driving the development of future intelligent societies. It highlights cutting-edge research and industrial innovations led by the International Web Intelligence Consortium, including Web Intelligence 3.0-based wisdom services, intelligent computing for brain big data, and advanced solutions for the prevention and treatment of psychiatric and neurocognitive disorders through intelligent health technologies. These initiatives address critical societal challenges and set the stage for the next generation of intelligent systems and services.
Short-Bio:
Ning Zhong received the Ph.D. degree from the University of Tokyo. He currently holds positions as the chairman of the Web Intelligence Consortium (WIC, wi-consortium.org), professor emeritus and visiting professor, and previously served as a professor in the Department of Life Science and Informatics at Maebashi Institute of Technology, Japan. His research interests focus on Web Intelligence (WI), Brain Informatics (BI), machine learning, data mining, intelligent health technologies, and intelligent systems. He serves as the founding editor-in-chief of the Web Intelligence journal (IOS Press), the editor-in-chief of the Brain Informatics journal (Springer Nature). He is a foreign fellow of the Engineering Academy of Japan (EAJ).
Plenary
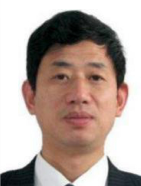
Dewen Hu
National University of Defense Technology, China
Title: Brain Cognitive Pattern Analysis and Brain-computer Interface
Abstract:
Firstly, I introduce the construction and verification of the whole human brain mapping parcellation. Secondly , I suggest some results on few-shot learning for diagnosis of neural and mental health. Finally, I will present some progress on brain-computer interface and applications.
Short-Bio:
Dewen Hu (Senior Member, IEEE) received the B.S. and M.S. degrees from Xi’an Jiaotong University, Xi’an, China, in 1983 and 1986, respectively, and the Ph.D. degree from the National University of Defense Technology, Changsha, China, in 1999. He is currently a Professor with the School of Intelligent Science and Technology, National University of Defense Technology. From 1995 to 1996, he was a Visiting Scholar with the University of Sheffield, Sheffield, U.K. His research interests include image processing, system identification and control, neural networks, and cognitive science.
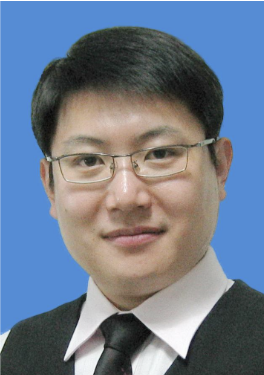
Dong Ming
Tianjin University, China
Title: Development and Challenges of Non-invasive Brain- Computer Interface
Abstract:
Brain-Computer interaction (BCI) represents the pinnacle of human-machine interaction and is an essential pathway for the integration of artificial intelligence into biological intelligence. The technique has emerged as a new favorite in the realm of brain science research, bearing significant implications for advancing modern medicine. This presentation initially provides an overview of BCI by summarizing the common developmental trends of both invasive and non-invasive types. Subsequently, the complete technological chain of BCI, including neural foundations, sensing methods, software and hardware systems, key technologies, and typical applications will be addressed. The presentation will also introduce the latest research progress of the neural engineering group of Tianjin University in the field and provides a summary of the developmental trends and future directions of BCI at the end.
Short-Bio:
Professor Dong Ming, Vice President of Tianjin University, Chair Professor. He was a winner of Distinguished Young Scholars of the National Nature Science Fund, National Special Support Plan for High-level Talents, young and middle-aged scientific and technological innovation leading talents of the Ministry of Science and Technology. He serves as member of the Science and Technology Committee of the Ministry of Education and holds prestigious positions including Director of the National Health and Medical Big Data Research Institute, Director of the Intelligent Medical Engineering Research Center at the Ministry of Education, and Executive Director of the Haihe Laboratory of Brain-Computer Interaction and Human-Machine Integration. He is also a Life Member of the International Functional Electrical Stimulation Society (IFESS), the editor in chief of the Journal of Intelligent Medicine, the Vice Chairman of the Chinese Society of Biomedical Engineering, and the Vice Chairman of the Brain-Computer Interface and Brain-like Intelligence Professional Committee at the China Standardization Association.

Qing Nie
University of California, Irvine
Department of Mathematics
Department of Developmental and Cell Biology
NSF-Simons Center for Multiscale Cell Fate Research
Title: Spatiotemporal Learning of High-dimensional Cell Fate
Abstract:
Cells make fate decisions in response to dynamic environments, and multicellular structures emerge from multiscale interplays among cells and genes in space and time. The recent single-cell genomics technology provides an unprecedented opportunity to profile cells for all their genes. While those measurements provide high-dimensional gene expression profiles for all cells, it requires fixing individual cells that lose many important spatiotemporal information. Is it possible to infer temporal relationships among cells from single or multiple snapshots? How to recover spatial interactions among cells, for example, cell-cell communication? In this talk I will present our newly developed computational tools that are mostly based on dynamical models and machine-learning methods, with a focus on inference and analysis of transitional properties of cells and cell-cell communication using both high-dimensional single-cell and spatial transcriptomics, as well as multi-omics data for some cases. Through their applications to various complex systems in development, regeneration, and diseases, we show the discovery power of such methods in addition to identifying areas for further method development for spatiotemporal analysis of single-cell data.
Short-Bio:
Dr. Qing Nie is a University of California Presidential Chair and a Distinguished Professor of Mathematics and Developmental & Cell Biology at University of California, Irvine. Dr. Nie is also a University of California Presidential Chair, and the director of the NSF-Simons Center for Multiscale Cell Fate Research jointly funded by NSF and the Simons Foundation – one of the four national centers on mathematics of complex biological systems. In research, he uses systems biology and data-driven methods to study complex biological systems with focuses on single-cell analysis, multiscale modeling, cellular plasticity, stem cells, embryonic development, and their applications to diseases. Dr. Nie has published more than 200 research articles, including several papers in Nature, Nature Methods, and Nature Machine Intelligence. In training, Dr. Nie has supervised more than 60 postdoctoral fellows and PhD students, with many of them working in academic institutions. Dr. Nie is a fellow of the American Association for the Advancement of Science (AAAS), American Physical Society (APS), Society for Industrial and Applied Mathematics (SIAM), and American Mathematical Society (AMS).
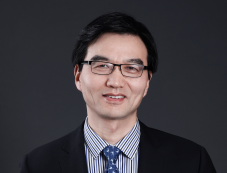
Dinggang Shen
School of BME, ShanghaiTech University, China
Shanghai United Imaging Intelligence Co., Ltd., China
Title: Artificial Intelligence for Whole Clinical Workflow: From Data Acquisition to Health/Disease
Abstract:
I will introduce our developed full-stack, full-spectrum Artificial Intelligence (AI, or deep learning) techniques for whole clinical workflow, from data acquisition to disease detection, follow-up, diagnosis, therapy, and outcome prediction. In particular, I will demonstrate some innovative technical development and implementation in scanners and clinical workflows, i.e., serving for fast MR, low-dose CT/PET acquisition, and clinical diagnosis/therapy.
Short-Bio:
Dinggang Shen is a Professor and a Founding Dean with School of Biomedical Engineering, ShanghaiTech University, Shanghai, China, and also a Co-CEO of United Imaging Intelligence (UII), Shanghai. He is a Fellow of IEEE, AIMBE, IAPR, and MICCAI. He was also a recipient of the Distinguished Investigator Award from The Academy for Radiological & Biomedical Imaging Research, USA (2019). He was Jeffrey Houpt Distinguished Investigator and a Full Professor (Tenured) with The University of North Carolina at Chapel Hill (UNC-CH), Chapel Hill, NC, USA, directing The Center of Image Analysis and Informatics, The Image Display, Enhancement, and Analysis (IDEA) Lab, and The Medical Image Analysis Core. Before that, he was also a tenure-track assistant professor in the University of Pennsylvanian (UPenn), and an Instructor in the Johns Hopkins University. His research interests include medical image analysis, computer vision, and pattern recognition. He has published more than 1600 peer-reviewed papers in the international journals and conference proceedings, with H-index 140 and over 85K citations. He serves as an Editor-in-Chief for Frontiers in Radiology, as well as an editorial board member for eight international journals. Also, he has served in the Board of Directors for MICCAI Society in 2012-2015, and was General Chair for MICCAI 2019.
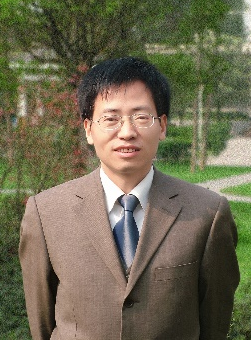
Dezhong Yao
University of Electronic Science and Technology, China
Title: Neuromodulation 3.0: brain-apparatus communication based neuromodulation
Abstract:
Neuromodulation is a rapidly developing field, with various new technologies for the treatment of various neurological and neuropsychiatric disorders, but it still suffers from a few big challenges such as the individual differences and effectiveness predictability. Therefore, it is an important issue to clarify the development routine of neuromodulation technology (NMT) to address these difficulties. Here based on the relation between NMT and subject, we suppose to divide NMT into three generations: (1) open-loop NMT where neuromodulation and subject are independent; (2) one closed-loop such as bidirectional brain-computer interface(BCI) based NMT where neuromodulation is brain state dependent; and (3) Multiple closed-loops such as using brain-physical instrument loop and brain-living organ loop simultaneously, i.e., brain-apparatus communication(BAC) based NMT where neuromodulation is dependent on both brain and body status.
Short-Bio:
He is a professor and the director of the “China-Cuba neurotechnology and brain-apparatus communication ‘one belt and one road’ joint Lab”, University of Electronic Science and Technology of China. He innovated the REST technique for zero EEG reference at infinity, and proposed the concept of ‘Brain-Apparatus Communication’ (BAC) to integrate brain-computer interface and psychosomatics. He has published 200+ peer reviewed publications including the recent monograph “ The physics and Mathematics of electroencephalogram”(2024,CRC). He is AIMBE Fellow, winner of the Roy John Award of ECNS, first class natural award of MOE and Huangjiasi award of CSBME.

James Zou
Stanford University, USA
Title: Al agents for biomedical discoveries
Abstract:
This talk will explore how generative Al agents can enable scientific discoveries. First, I will discuss how generative Al can expand researchers' creativity by designing and experimentally validating new small-molecule drugs. Next, I'll introduce the Virtual Lab——a collaborative team of Al agents conducting in silico research meetings, supported by real-world experiments, to design new therapeutics.
Short-Bio:
James Zou is an associate professor of Biomedical Data Science, CS and EE at Stanford University. He works on advancing the foundations of ML and in-depth scientific and clinical applications. Many of his innovations are widely used in tech and biotech industries. He has received a Sloan Fellowship, an NSF CAREER Award, two Chan-Zuckerberg nvestigator Awards, a Top Ten Clinical Achievement Award, several best paper awards, and faculty awards from Google, Amazon, and Adobe. His research has also been profiled in popular press including the NY Times, WSJ, and WIRED.